A popular criticism of artificial intelligence recently has been that it uses a staggering amount of energy, echoing similar arguments against cryptocurrency.
In both cases, these claims are usually advanced by people who are already biased against the technology for one reason or another, so we should be skeptical. But neither do we want to dismiss the claims just because of the source. But I have never put in the effort to seriously fact-check either claim.
Fortunately, @urusan@fosstodon.org has collected some sources for us on the subject in a thread on the Fediverse.
With his permission, I’m republishing his posts (edited only to add Internet Archive permalinks for the references) here.
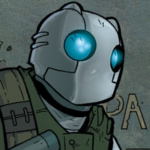
Thread: “AI, Energy Consumption”
I was watching a YouTube video and they quoted that ChatGPT/GPT-4 uses 300Wh per request. This was so beyond reasonable that I had to go check and what I found was a rabbit hole.
It turns out that there’s very little evidence that AI is actually using the truly staggering amounts of electricity that have been widely reported.
Yes, even in the giant companies at the center of the hype bubble (with one possible recent exception, because Google is being self-destructive).
Let’s review the older evidence that got the whole “AI is using a small country’s worth of electricity” ball rolling.
First of all, I tracked the 300Wh per request figure to this Stack Overflow https://ai.stackexchange.com/a/39018 [ARCHIVE]
It’s a speculative, worst-case back of the envelope estimate based on a quote by Sam Altman saying it cost them single digit cents per chat to run ChatGPT, so $0.09 in electricity.
It’s pessimistic speculation based on hearsay, so that’s not a good source.
So then I looked into what kind of claim was being made more generally and traced various articles back to this piece in the New Yorker: https://www.newyorker.com/news/daily-comment/the-obscene-energy-demands-of-ai [ARCHIVE]
This (2024) article doesn’t provide a source for its direct estimates (though it does point to the root of the idea elsewhere in the article). It says “It’s been estimated that ChatGPT” gets 200M daily requests and spends 0.5M kWh daily. This is actually a pretty good estimate, but it’s not close to country-scale.
The New Yorker article mentions Alex de Vries, an economics grad student that published this paper https://asociace.ai/wp-content/uploads/2023/10/ai-spotreba.pdf [ARCHIVE] and cross-posted it on his blog about cryptocurrency sustainability https://digiconomist.net/powering-ai-could-use-as-much-electricity-as-a-small-country/ [ARCHIVE]
along with all the news releases for his paper.
The key claim is that in 4 years Nvidia could potentially deliver enough AI hardware to rival the power consumption of cryptocurrency and small countries if the AI hype continues to ramp up and all capacity is used
I don’t think de Vries is unreasonable, he’s done a good job collecting most of the available figures, and the scenario he outlines isn’t completely unrealistic.
I also think his concern is a good one, it would be undesirable to spend this much electricity on AI, especially on wasteful replacements for systems that work fine without AI.
He also predicted Google’s bad decisions about a year before it actually happened.
That said, de Vries is definitely thinking about this from the cryptocurrency-interested economist perspective.
This scenario is basically the scenario where the AI hype bubble goes “to the moon”. I don’t think it will actually get that far as the limitations of AI start to become more apparent.
Also, while his point about Jevon’s paradox eating up all the efficiency gains isn’t a bad one, it does assume we keep finding new (or unlimited scale) applications for AI.
That said, de Vries is definitely thinking about this from the cryptocurrency-interested economist perspective.
This scenario is basically the scenario where the AI hype bubble goes “to the moon”. I don’t think it will actually get that far as the limitations of AI start to become more apparent.
Also, while his point about Jevon’s paradox eating up all the efficiency gains isn’t a bad one, it does assume we keep finding new (or unlimited scale) applications for AI.
That said, de Vries is definitely thinking about this from the cryptocurrency-interested economist perspective.
This scenario is basically the scenario where the AI hype bubble goes “to the moon”. I don’t think it will actually get that far as the limitations of AI start to become more apparent.
Also, while his point about Jevon’s paradox eating up all the efficiency gains isn’t a bad one, it does assume we keep finding new (or unlimited scale) applications for AI.
I was just looking at this BBC article and they just focus on the 4 years in the future hypothetical and don’t make any attempt to estimate the current usage for comparison. https://www.bbc.com/news/technology-67053139 [ARCHIVE]
It’s clear where people got the idea that it’s country-scale when it’s not…
That said, recent news is that Google’s Gemini Ultra cost $191 million to train (and further back GPT-4 cost $78 million to train). https://hai.stanford.edu/news/inside-new-ai-index-expensive-new-models-targeted-investments-and-more [ARCHIVE]
A worst case estimate would be that all that was electricity, in which case Gemini Ultra cost 191M$ * 0.10$/kWh = 1,910 GWh to train, so about 1.6% of the Netherlands yearly electricity budget.
GPT-4’s training would have been 780 GWh or 0.6% of the Netherlands, or about 4 years of their estimated operating cost.
That said, Google’s Gemini Ultra is also the harbinger of the end of the AI bubble.
By their own admission, they only got small benefits from a model that cost 2x more to train and is probably much larger: https://blog.google/technology/ai/google-gemini-ai/#performance [ARCHIVE]
So either Google really sucks at AI or we’re on the downslope of the GenAI capabilities S-curve.
Researchers might be able to figure out something to keep the train running, but the days of “just keep scaling and it’ll get way better” are over.
Really, that trend was over with GPT-4, and they knew and were hiding that weakness this whole time…
Regardless though, we’re not realistically going to see the country-scale AI electrical consumption deployed 4 years from now scenario. The bubble will pop long before then…or if it does continue it’ll be kept afloat by efficiency and engineering improvements, not just going big and energy hungry.
Additional Sub-Thread “AI”
(Some speculation on the future of Generative AI)
Even though I expect that the S-curve is slowing down, I do think GenAI specifically and AI in general have a bright future, it’s just different from the vision pushed by Silicon Valley and the hype bubble.
They’re hoping to build a superintelligent general AI that can do it all. Some want this for futurism reasons and others want to own it. It’s not a realistic goal however, for a single unitary intelligence know everything and be good at everything.
I anticipate that the real path forward with AI is in building collective intelligence out of connected collections of smaller, specialized models and traditional systems.
This isn’t to say that only small, specialized models are reasonable. It’s just that it seems like we’ve found a scale sweet spot, where we have gotten most of the benefits from scale and going much bigger is wasteful.
The general models are useful as a starting point for training specialized models.
I also think it’s better to think of these GenAI systems as more like language adapters or language processing centers.
That’s their primary value, processing language and connecting us to otherwise inaccessible systems or phenomena.
They also have potential value in dealing with chaos and large, complex problems which traditional methods struggle with.
In his permission grant, Urusan says this can be treated as CC-0/Public Domain, but he’d appreciate credit.